Program
Sun 16 Oct |
International Conference Hall I |
International Conference Hall II |
International Conference Hall III |
||
13:00 | 14:30 |
Tutorial 1Aapo Hyvärinen | ||||
14:30 | 16:00 |
Tutorial 3Nikola Kasabov | Tutorial 4Okito Yamashita |
|||
16:30 | 18:30 | Best Student Paper Awards Presentation | ||||
18:30 | 20:30 |
Welcome Reception |
||||
The conference venue, Kyoto University Clock Tower Centennial Hall, is not open until 9:00. | |||||
Mon 17 Oct |
Clock Tower Centennial Hall | ||||
9:20 | 9:30 |
Openning Remark
Akira Hirose, General Chair |
||||
9:30 | 10:20 |
Plenary 1 Kunihiko Fukushima |
||||
International Conference Hall I |
International Conference Hall III |
Conference Room III | Conference Room IV | ||
10:40 | 12:20 |
Special Session 1Deep and Reinforcement Learning |
Big data analysis | Neural data analysis | Robotics and control | |
13:20 | 15:00 |
Special Session 2Bio-Inspired / Energy-Efficient Information Processing: Theory, Systems, Devices |
Special Session 3Whole Brain Architecture: Toward a Human Like General Purpose Artificial Intelligence |
Neurodynamics 1 | Bioinformatics | |
15:20 | 17:00 |
Neurodynamics 2 | Biomedical engineering Cialis 20 mg | |||
Tue 18 Oct |
Clock Tower Centennial Hall | ||||
9:30 | 10:20 |
Plenary 2 Irwin King |
||||
International Conference Hall I |
International Conference Hall III |
Conference Room III | Conference Room IV | ||
10:40 | 12:20 |
Workshop 1 webData Mining and Cybersecurity WorkshopInvited Talks: |
Neuromorphic hardware | Machine learning 1 | Sensory perception | |
13:20 | 15:00 |
Pattern recognition
Invited Talk: |
Machine learning 2 | Social networks | ||
15:20 | 17:20 |
Machine learning 3 | ||||
International Conference Hall II | |||||
17:20 | 19:00 |
Poster 1 |
||||
Wed 19 Oct |
Clock Tower Centennial Hall | ||||
9:30 | 10:20 |
Plenary 3 Mitsuo Kawato |
||||
International Conference Hall I |
International Conference Hall III |
Conference Room III | Conference Room IV | ||
10:40 | 12:20 |
Brain-machine interface | Computer vision 1 | Machine learning 4 | Time series analysis | |
13:00 | 14:00 |
JNNS Meeting |
||||
14:00 | 15:40 |
Special Session 4Data-Driven Approach for Extracting Latent Features from Multi-Dimensional Data |
Computer vision 2 | Machine learning 5 |
Special Session 5Topological and Graph Based Clustering MethodsTutorial: |
|
16:00 | 17:40 |
Computer vision 3 | Machine learning 6 | |||
International Conference Hall II | |||||
17:40 | 19:20 |
Poster 2 |
||||
Thu 20 Oct |
Clock Tower Centennial Hall | ||||
9:30 | 10:20 |
Plenary 4 Sebastian Seung |
||||
International Conference Hall I |
International Conference Hall II |
International Conference Hall III |
Conference Room III | Conference Room IV | |
10:40 | 12:20 |
Reinforcement learning | Applications |
Computational intelligence 1 |
Data mining 1 | Deep neural networks 1 |
13:20 | 15:00 |
Workshop 2 webNovel Approaches of Systems Neuroscience to Sports and RehabilitationSpecial Talk: |
Machine learning 7 |
Computational intelligence 2 |
Data mining 2 | Deep neural networks 2 |
15:20 | 17:00 |
Computer vision 4 |
Computational intelligence 3 |
Data mining 3 | Deep neural networks 3 | |
Kyoto Hotel Okura [Information] | |||||
17:30 | 18:30 |
Tea Ceremony & Maiko Greetings |
||||
18:30 | 21:30 |
Banquet & APNNS Regular Meeting of Members |
||||
Fri 21 Oct |
|||||
9:00 | |
Technical Tour to ATR |
||||
Oral presentationAll presentations must be in English. Each presenter will have 15 minutes for presentation and 5 minutes for questions. The projector equips VGA and HDMI, so please bring an appropriate adaptor for your computer. Please arrive to your session early for testing your presentation (especially if you have audio or video). Note we provide a PC with each projector where PowerPoint and Acrobat Reader run on Windows 7. Poster presentationAll posters must be written in English and be printed by the authors in advance. Each poster should include the title as well as the names and affiliations of the authors. Posters must not exceed A0 portrait size (H2100 x W900 mm). |
Plenary Talks
9:30-10:20 Mon 17 Oct
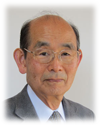
Kunihiko Fukushima
Senior Research Scientist,Fuzzy Logic Systems Institute,
Fukuoka, Japan
9:30-10:20 Tue 18 Oct

Irwin King
Professor and Associate Dean,The Chinese University of Hong Kong, Hong Kong, China
9:30-10:20 Wed 19 Oct
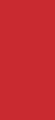
Mitsuo Kawato
Director,ATR Brain Information Communication Research Laboratory Group,
Kyoto, Japan
9:30-10:20 Thu 20 Oct
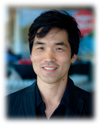
Sebastian Seung
Professor,Princeton Neuroscience Institute and Computer Science Department,
Princeton, US
Plenary 1
Clock Tower Centennial Hall
Deep CNN Neocognitron for Visual Pattern Recognition
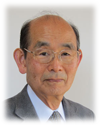
Senior Research Scientist, Fuzzy Logic Systems Institute (Iizuka, Fukuoka, Japan)
URL: http://personalpage.flsi.or.jp/fukushima/index-e.html
Recently, deep convolutional neural networks (deep CNN) have become very popular in the field of visual pattern recognition. The neocognitron, which was first proposed by Fukushima (1979), is a network classified to this category. Its architecture was suggested by neurophysiological findings on the visual systems of mammals. It is a hierarchical multi-layered network. It acquires the ability to recognize visual patterns robustly through learning.
Although the neocognitron has a long history, improvements of the network are still continuing. This talk discusses the recent neocognitron focusing on differences from the conventional deep CNN. For training intermediate layers of the neocognitron, the learning rule called AiS (Add-if-Silent) is used. Under the AiS rule, a new cell is generated if all postsynaptic cells are silent in spite of non-silent presynaptic cells. The generated cell learns the activity of the presynaptic cells in one-shot. Once a cell is generated, its input connections do not change any more. Thus the training process is very simple and does not require time-consuming repetitive calculation. In the deepest layer, a method called Interpolating-Vector is used for classifying input patterns based on the features extracted in the intermediate layers.
Biography
Kunihiko Fukushima received a B.Eng. degree in electronics in 1958 and a PhD degree in electrical engineering in 1966 from Kyoto University, Japan. He was a professor at Osaka University from 1989 to 1999, at the University of Electro-Communications from 1999 to 2001, at Tokyo University of Technology from 2001 to 2006; and a visiting professor at Kansai University from 2006 to 2010. Prior to his Professorship, he was a Senior Research Scientist at the NHK Science and Technical Research Laboratories. He is now a Senior Research Scientist at Fuzzy Logic Systems Institute (part-time position), and usually works at his home in Tokyo.
He received the Achievement Award and Excellent Paper Awards from IEICE, the Neural Networks Pioneer Award from IEEE, APNNA Outstanding Achievement Award, Excellent Paper Award from JNNS, INNS Helmholtz Award, and so on. He was the founding President of JNNS (the Japanese Neural Network Society) and was a founding member on the Board of Governors of INNS (the International Neural Network Society). He is a former President of APNNA (the Asia-Pacific Neural Network Assembly).
Plenary 2
Clock Tower Centennial Hall
Recent Developments in Online Learning for Big Data Applications

Department of Computer Science & Engineering
The Chinese University of Hong Kong
URL: http://www.cse.cuhk.edu.hk/irwin.king
As data generated from sciences, business, governments, etc. are reaching petabyte or even exabyte at an alarming rate, theories, models, and applications in online learning is becoming important in machine learning to process a large amount of streaming data effectively and efficiently. Recently, a number of online learning algorithms have been proposed to tackle the issues of ultra-dimension and high imbalance among the data. In this talk, we focus on new developments of online learning technologies in both theory and applications. Important topics including online boosting, online learning for sparse learning models, and distributed online algorithms, etc. will be discussed. Moreover, some of our recent works such as online learning for multi-task feature selection, imbalanced data, online dictionary learning, etc., will also be presented to demonstrate how online learning approaches can effectively handle streaming big data.
Biography
Irwin King is the Associate Dean (Education) of the Engineering Faculty and Professor in the Department of Computer Science and Engineering, The Chinese University of Hong Kong. He is also the Director of Rich Media and Big Data Key Laboratory at the Shenzhen Research Institute. His research interests include machine learning, social computing, Big Data, data mining, and multimedia information processing. In these research areas, he has over 225 technical publications in journals and conferences.
Prof. King is the Book Series Editor for Social Media and Social Computing with Taylor and Francis (CRC Press). He is also an Associate Editor of the Neural Network Journal and ACM Transactions on Knowledge Discovery from Data (ACM TKDD). Currently, he is a member of the Board of Governors of INNS and a Vice-President and Governing Board Member of APNNA. He also serves INNS as the Vice-President for Membership in the Board of Governors. Moreover, he is the General Chair of WSDM2011, General Co-Chair of RecSys2013, ACML2015, and in various capacities in a number of top conferences such as WWW, NIPS, ICML, IJCAI, AAAI, etc.
Prof. King is Associate Dean (Education), Faculty of Engineering and Professor at the Department of Computer Science and Engineering, The Chinese University of Hong Kong. He is also Director of the Shenzhen Key Laboratory of Rich Media and Big Data. He received his B.Sc. degree in Engineering and Applied Science from California Institute of Technology, Pasadena and his M.Sc. and Ph.D. degree in Computer Science from the University of Southern California, Los Angeles. He was on leave to AT&T Labs Research on special projects and also taught courses at UC Berkeley on Social Computing and Data Mining. Recently, Prof. King has been an evangelist in the use of education technologies in eLearning for the betterment of teaching and learning.
Plenary 3
Clock Tower Centennial Hall
DecNef: tool for revealing brain-mind causal relation
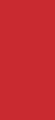
Director,
ATR Brain Information Communication Research Laboratory Group,
Kyoto, Japan URL:
http://www.cns.atr.jp/%7Ekawato/
One of the most important hypotheses in neuroscience is that human mind is caused by a specific spatiotemporal activity pattern in the brain. Here, human mind includes perception, emotion, movement control, action plan, attention, memory, metacognition and consciousness. This is a central hypothesis for computational and system neuroscience, but has never been experimentally examined. One of the reasons for this failure is that most neuroscientists including myself, from the beginning, gave up the possibility to experimentally control spatiotemporal brain activity in humans. Decoded neurofeedback (DecNef) is a novel method to fulfill this requirement by combining real-time fMRI neurofeedback, decoding of multi-voxel patterns by sparse machine learning algorithms, and reinforcement learning by human participants while avoiding “curse of dimensionality”. Kazuhisa Shibata and colleagues demonstrated that V1/V2 patterns can be controlled for specific orientation information (Science, 2011). In the past 5 years, we succeeded to control color in V1/V2 (Amano et al., Curr Biol, 2016), facial preference in the cingulate cortex (Shibata et al., PLoS Biol, 2016), perceptual confidence for motion discrimination in dorsolateral prefrontal cortex and inferior parietal lobule (Cortese et al., under review, 2016), and reduction of fear memory in V1/V2 (Koizumi et al., under revision, 2016) by DecNef. Furthermore, DecNef was shown to be capable of changing brain dynamics for therapeutic purposes (central pain by Yanagisawa, Saito et al., obsessive and compulsive disorder by Sakai and Tanaka).
Biography
Mitsuo Kawato received the B.S. degree in physics from Tokyo University in 1976 and the M.E. and Ph.D. degrees in biophysical engineering from Osaka University in 1978 and 1981, respectively. From 1981 to 1988, he was a faculty member and lecturer at Osaka University. From 1988, he was a senior researcher and then a supervisor in ATR Auditory and Visual Perception Research Laboratories. In 1992, he became department head of Department 3, ATR Human Information Processing Research Laboratories. From 2003, he has been Director of ATR Computational Neuroscience Laboratories. For the last 30 years, he has been working in computational neuroscience.
Plenary 4
Clock Tower Centennial Hall
Neural nets and the connectome
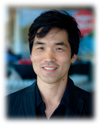
Neuroscience Institute and Computer Science Dept. Princeton University
http://seunglab.org
Since the dawn of AI in the 1950s, inspiration from the brain has helped researchers make computers more intelligent. In turn, AI is now helping to accelerate research on understanding the brain. A prime example is the application of artifical neural networks to brain images from 3D electron microscopy. It is starting to become possible to reconstruct the brain's wiring diagram, or "connectome." This approach has already yielded discoveries concerning the first steps of visual perception in the retina. Further progress is expected to yield connectomic information from the cerebral cortex, regarded by many neuroscientists as the brain region most crucial for human intelligence.
Biography
Sebastian Seung is Anthony B. Evnin Professor in the Neuroscience Institute and Department of Computer Science at Princeton University. Seung has done influential research in both computer science and neuroscience. Over the past decade, he has helped pioneer the new field of connectomics, developing new computational technologies for mapping the connections between neurons. His lab created EyeWire.org, a site that has recruited over 200,000 players from 150 countries to a game to map neural connections. His book Connectome: How the Brain's Wiring Makes Us Who We Are was chosen by the Wall Street Journal as Top Ten Nonfiction of 2012. Before joining the Princeton faculty in 2014, Seung studied at Harvard University, worked at Bell Laboratories, and taught at the Massachusetts Institute of Technology. He is External Member of the Max Planck Society, and winner of the 2008 Ho-Am Prize in Engineering.
Tutorials
Tutorial 1
International Conference Hall I
Non-Gaussian machine learning: From ICA to unsupervised deep learning
Aapo HyvärinenUniversity Of Helsinki, Finland
Abstract: Non-Gaussianity is a key concept in several machine learning techniques. In the 1990's, its importance was understood in the framework of independent component analysis (ICA). Application to Bayesian networks was proposed in 2006 as the Linear Non-Gaussian Acyclic Model (LiNGAM). Related models have been developed for time series as well, for example as non-Gaussian autoregressive models, or non-Gaussian state-space models. While the theory for such linear models is well understood by now, extending the theory to nonlinear models is an important question in current and future research. In particular, some recent efforts in deep unsupervised learning can be seen as attempts to accomplish such a nonlinear extension, but they often resort to heuristic criteria instead of justified probabilistic models. I will discuss some of my very recent results extending the ICA framework to nonlinear models, in order to accomplish principled nonlinear (deep) feature extraction.
Biography
Aapo Hyvärinen studied undergraduate mathematics at the universities of Helsinki (Finland), Vienna (Austria), and Paris (France), and obtained a Ph.D. degree in Information Science at the Helsinki University of Technology in 1997. After post-doctoral work at the Helsinki University of Technology, he moved to the University of Helsinki in 2003, where he is currently Professor of Computer Science, especially Machine Learning. Aapo Hyvarinen is the main author of the books "Independent Component Analysis" (2001) and "Natural Image Statistics" (2009), and author or coauthor of more than 200 scientific articles. He is Action Editor at the Journal of Machine Learning Research and Neural Computation, Editorial Board Member in Foundations and Trends in Machine Learning, as well as Contributing Faculty Member of Faculty of 1000 Prime. His current work concentrates on applications of unsupervised machine learning methods to neuroscience.
Tutorial 3
International Conference Hall I
Deep Learning, Spiking Neural Networks and Evolving Spatio-Temporal Data Machines
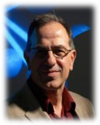
Knowledge Engineering and Discovery Research Institute (KEDRI),
Auckland University of Technology,
[email protected], www.kedri.aut.ac.nz
The current development of the third generation of artificial neural networks - the spiking neural networks (SNN) along with the technological development of highly parallel neuromorphic hardware systems of millions of artificial spiking neurons as processing elements, makes it possible to model complex data streams in a more efficient, brain-like way [1,2].
The tutorial first presents some principles of SNN and of deep learning in SNN. It introduces a recently proposed evolving SNN architecture called NeuCube. NeuCube was first proposed for brain data modelling [3,4]. It was further developed as a general purpose SNN development system for the creation and testing of temporal or spatio/spectro temporal data machines (STDM) to address challenging data analysis and modelling problems [5,11]. A version of the NeuCube development system is available free from: http://www.kedri.aut.ac.nz/neucube/, along with papers and case study data.
Then the tutorial introduces a methodology for the design and implementation of STDM based on SNN (STDM) for deep learning and for predictive data modelling of temporal or spatio-/spectro temporal data [5,11]. A STDM has modules for: preliminary data analysis, data encoding into spike sequences, unsupervised learning of temporal or spatio-temporal patterns, classification, regression, prediction, optimisation, visualisation and knowledge discovery. A STDM can be used to predict early and accurately events and outcomes through the ability of SNN to be trained to spike early, when only a part of a new pattern is presented as input data. The methodology is illustrated on benchmark data with different characteristics, such as: financial data streams; brain data for brain computer interfaces; personalised and climate date for individual stroke occurrence prediction [6]; ecological and environmental disaster prediction, such as earthquakes. The talk discusses implementation on highly parallel neuromorphic hardware platforms such as the Manchester SpiNNaker [7] and the ETH Zurich chip [8,9]. These STDM are not only significantly more accurate and faster than traditional machine learning methods and systems, but they lead to a significantly better understanding of the data and the processes that generated it. New directions for the development of SNN and STDM are pointed towards a further integration of principles from the science areas of computational intelligence, bioinformatics and neuroinformatics and new applications across domain areas [10,11].
References- EU Marie Curie EvoSpike Project (Kasabov, Indiveri): http://ncs.ethz.ch/projects/EvoSpike/
- Schliebs, S., Kasabov, N. (2013). Evolving spiking neural network-a survey. Evolving Systems, 4(2), 87-98.
- Kasabov, N. (2014) NeuCube: A Spiking Neural Network Architecture for Mapping, Learning and Understanding of Spatio-Temporal Brain Data, Neural Networks, 52, 62-76.
- Kasabov, N., Dhoble, K., Nuntalid, N., Indiveri, G. (2013). Dynamic evolving spiking neural networks for on-line spatio- and spectro-temporal pattern recognition. Neural Networks, 41, 188-201.
- Kasabov, N. et al (2016) A SNN methodology for the design of evolving spatio-temporal data machines, Neural Networks, 2016.
- Kasabov, N., et al. (2014). Evolving Spiking Neural Networks for Personalised Modelling of Spatio-Temporal Data and Early Prediction of Events: A Case Study on Stroke. Neurocomputing, 2014.
- Furber, S. et al (2012) Overview of the SpiNNaker system architecture, IEEE Trans. Computers, 99.
- Indiveri, G., Horiuchi, T.K. (2011) Frontiers in neuromorphic engineering, Frontiers in Neuroscience, 5, 2011.
- Scott, N., N. Kasabov, G. Indiveri (2013) NeuCube Neuromorphic Framework for Spatio-Temporal Brain Data and Its Python Implementation, Proc. ICONIP 2013, Springer LNCS, 8228, pp.78-84.
- Kasabov, N. (ed) (2014) The Springer Handbook of Bio- and Neuroinformatics, Springer.
- Kasabov, N (2016) Spiking Neural Networks and Evolving Spatio-Temporal Data Machines, Springer, 2016
Biography
Professor Nikola Kasabov is Fellow of IEEE, Fellow of the Royal Society of New Zealand and DVF of the Royal Academy of Engineering, UK. He is the Director of the Knowledge Engineering and Discovery Research Institute (KEDRI), Auckland. He holds a Chair of Knowledge Engineering at the School of Computing and Mathematical Sciences at Auckland University of Technology. Kasabov is a Past President and Governor Board member of the International Neural Network Society (INNS) and also of the Asia Pacific Neural Network Society (APNNS). He is a member of several technical committees of IEEE Computational Intelligence Society and a Distinguished Lecturer of the IEEE CIS (2012-2014). He is a Co-Editor-in-Chief of the Springer journal Evolving Systems and serves as Associate Editor of Neural Networks, IEEE TrNN, -Tr CDS, -TrFS, Information Science, Applied Soft Computing and other journals. Kasabov holds MSc and PhD from the TU Sofia, Bulgaria. His main research interests are in the areas of neural networks, intelligent information systems, soft computing, bioinformatics, neuroinformatics. He has published more than 600 publications that include 15 books, 180 journal papers, 80 book chapters, 28 patents and numerous conference papers. He has extensive academic experience at various academic and research organisations in Europe and Asia, including: TU Sofia, University of Essex, University of Otago, Advisor- Professor at the Shanghai Jiao Tong University, Visiting Professor at ETH/University of Zurich. Prof. Kasabov has received the APNNA ‘Outstanding Achievements Award’, the INNS Gabor Award for ‘Outstanding contributions to engineering applications of neural networks’, the EU Marie Curie Fellowship, the Bayer Science Innovation Award, the APNNA Excellent Service Award, the RSNZ Science and Technology Medal, and others. He has supervised to completion 38 PhD students. More information of Prof. Kasabov can be found on the KEDRI web site: http://www.kedri.aut.ac.nz.
Tutorial 4
International Conference Hall III
Analysis Methods for Understanding Human Brain Activities
Okito Yamashita,Neural Information Analysis Laboratories, ATR [email protected]
Since ancient times, it has remained a mystery how our brain generates our mind and intelligence. Recent advancement of brain measurement and data analysis allows for addressing this long-standing question. In the field of human brain science, brain activities related to behaviors, perception and higher cognitions are investigated using non-invasive measurements such as fMRI, magneto-enchepharography (MEG) and electro-enchepharography (EEG). These methods have been employed to comprehensively describe macro-scale organization of human brain functions such as functional brain mapping [1] and human connectome [2], [3]. Human brain imaging data is typically low quality and high dimensional. Combinations of signal processing, image processing and machine learning methods are indispensable to discover meaningful patterns hidden in high dimensional multivariate data.
In this tutorial, I review several studies using human brain imaging methods with particular focus on analysis methodological aspects. The first half focuses on analysis of fMRI data including functional brain mapping, decoding studies [4]–[6], and recent human connectome studies [7]. All of these studies take advantages of whole-brain measurement and/or high-spatial resolution (millimeter) of blood-oxygen-level dependent (BOLD) signals to understand spatial organization of human brain. The latter half focuses on analysis of MEG data which is magnetic field measurement generated by population neuronal activities on the brain. MEG has high temporal resolution (millisecond), allowing for investigation of human brain dynamics in behavioral time scale which cannot be done with fMRI. One of big challenges of MEG data analysis is to reconstruct brain activities from MEG measurements outside the head, which is an inverse problem referred to as the source localization problem. We have been attempting to solve the problem by multi-data integration approach. I will introduce series of studies in our laboratories including the high-spatio-temporal source imaging by MEG-fMRI integration with the hierarchical Bayesian model [8]–[10] and whole-brain network dynamics identification with the high dimensional state space methods [11]–[13].
References- R. S. J. Frackowiak, K. J. Friston, C. Frith, R. Dolan, C. J. Price, S. Zeki, J. Ashburner, and W. D. Penny, Human Brain Function, 2nd ed. Academic Press, 2003.
- O. Sporns, G. Tononi, and R. Kötter, “The human connectome: A structural description of the human brain.,” PLoS Comput. Biol., vol. 1, no. 4, p. e42, Sep. 2005.
- S. M. Smith, C. F. Beckmann, J. Andersson, E. J. Auerbach, J. Bijsterbosch, G. Douaud, E. Duff, D. a Feinberg, L. Griffanti, M. P. Harms, M. Kelly, T. Laumann, K. L. Miller, S. Moeller, S. Petersen, J. Power, G. Salimi-Khorshidi, A. Z. Snyder, A. T. Vu, M. W. Woolrich, J. Xu, E. Yacoub, K. Uğurbil, D. C. Van Essen, and M. F. Glasser, “Resting-state fMRI in the Human Connectome Project.,” Neuroimage, vol. 80, pp. 144–68, Oct. 2013.
- Y. Kamitani and F. Tong, “Decoding the visual and subjective contents of the human brain.,” Nat. Neurosci., vol. 8, no. 5, pp. 679–85, May 2005.
- Y. Miyawaki, H. Uchida, O. Yamashita, M. Sato, Y. Morito, H. C. Tanabe, N. Sadato, and Y. Kamitani, “Visual image reconstruction from human brain activity using a combination of multiscale local image decoders.,” Neuron, vol. 60, no. 5, pp. 915–29, Dec. 2008.
- K. N. Kay, T. Naselaris, R. J. Prenger, and J. L. Gallant, “Identifying natural images from human brain activity.,” Nature, vol. 452, no. 7185, pp. 352–5, Mar. 2008.
- I. Tavor, O. P. Jones, R. B. Mars, S. M. Smith, T. E. Behrens, and S. Jbabdi, “Task-free MRI predicts individual differences in brain activity during task performance,” Science (80-. )., vol. 352, no. 6282, pp. 216–220, Apr. 2016.
- T. Yoshioka, K. Toyama, M. Kawato, O. Yamashita, S. Nishina, N. Yamagishi, and M. Sato, “Evaluation of hierarchical Bayesian method through retinotopic brain activities reconstruction from fMRI and MEG signals.,” Neuroimage, vol. 42, no. 4, pp. 1397–413, Oct. 2008.
- A. Toda, H. Imamizu, M. Kawato, and M. Sato, “Reconstruction of two-dimensional movement trajectories from selected magnetoencephalography cortical currents by combined sparse Bayesian methods.,” Neuroimage, vol. 54, no. 2, pp. 892–905, Jan. 2011.
- M. Sato, T. Yoshioka, S. Kajihara, K. Toyama, N. Goda, K. Doya, and M. Kawato, “Hierarchical Bayesian estimation for MEG inverse problem.,” Neuroimage, vol. 23, no. 3, pp. 806–26, Nov. 2004.
- M. Fukushima, O. Yamashita, T. R. Knösche, and M. Sato, “MEG source reconstruction based on identification of directed source interactions on whole-brain anatomical networks,” Neuroimage, vol. 105, pp. 408–427, 2015.
- O. Yamashita, A. Galka, T. Ozaki, R. Biscay, and P. A. Valdés-sosa, “Recursive penalized least squares solution for dynamical inverse problems of EEG generation.,” Hum. Brain Mapp., vol. 21, no. 4, pp. 221–35, Apr. 2004.
- A. Galka, O. Yamashita, T. Ozaki, R. Biscay, and P. A. Valdés-sosa, “A solution to the dynamical inverse problem of EEG generation using spatiotemporal Kalman filtering.,” Neuroimage, vol. 23, no. 2, pp. 435–53, Oct. 2004.
Biography
He has received M.A. in Engineering from Department of Mathematical Engineering and Information Physics, The University of Tokyo in 2001 and has received Ph.D. in Statistics from Department of Statistical Science, Graduate University for Advanced Studies in 2004. Then he joined Computational Neuroscience Laboratories, ATR in Kyoto as a researcher and promoted to a senior researcher of Neural Information Analysis Laboratories in 2010 and has been a head of department of Computational Brain Imaging since 2013. His research interest is to develop novel data analysis methodology for human brain science with multi-modal data integration approach. His research topics include MEG/EEG source localization problem, fMRI decoding, brain computer interface, diffusion optical tomography and dynamical system identification. He has been involved in two open-source code projects VBMEG (http://vbmeg.atr.jp/) and Sparse estimation toolbox (http://www.cns.atr.jp/cbi/sparse_estimation/index.html) both of which count more than ten thousands downloads.
Canceled
Tutorial 2
International Conference Hall III
The Use of Robotic Technology and Control Theory to Explore Brain Function and Dysfunction
Stephen Scott,GSK-CIHR Chair in Neuroscience,
Centre for Neuroscience Studies, Department of Biomedical and Molecular Sciences, Queen’s University
We take for granted the ease that we can move and interact in the environment, as it takes little conscious effort to reach out to grab an object of interest or use a fork to pick up food. The present talk will describe two lines of research that exploit the use of robotic technology to quantify upper limb motor function and dysfunction. My basic research program explores the neural, mechanical and behavioural aspects of sensorimotor function. Inspired by optimal control theory, we have performed a series of studies that illustrate the surprising sophistication of the human motor system to rapidly respond to small mechanical disturbances of the arm during goal-directed motor actions. The ability of robots to quantify motor performance makes them also potentially useful as a next generation technology for neurological assessment. Most assessment scales for sensorimotor function are subjective in nature with relatively coarse rating systems, reflecting that it is difficult for even experienced observers to discriminate consistently small changes in performance using only the naked eye. I will discuss a number of novel robot-based tasks that we’ve developed to assess brain function in subjects with stroke, highlighting the complex patterns of sensory, motor and cognitive deficits that can be quantified with this technology.
Biography
Received B.A.Sc and M.A.Sc in Systems Design Engineering from the University of Waterloo (supervisor Dr. D.A. Winter), and a Ph.D in Physiology from Queen’s University (supervisor, G. Loeb). He completed his post-doctoral training at the University of Montreal from 1993 to 1995 under the supervision of Dr. John Kalaska. He presently holds the GSK-CIHR Chair in Neuroscience in the Centre for Neuroscience Studies at Queen’s University. His research program includes technology development, basic research on voluntary motor control and clinical research on the use of robots for neurological assessment. He has published over 110 refereed journal articles and given over 160 invited talks. He is the inventor of the KINARM robot and is actively involved in the continued development of advanced technologies for use in basic and clinical research. He is Co-Founder and Chief Scientific Officer of BKIN Technologies that commercializes the KINARM robot and associated technologies.
Special Session 1
International Conference Hall I
Deep and Reinforcement Learning
ProgramOrganizers:
Abdulrahman Altahhan, Coventry University, UK
Vasile Palade, Coventry University, UK
Scope:
Deep Learning has been under the focus of neural network research and industrial communities due to its proven ability to scale well into difficult problems and due to its performance breakthroughs over other architectural and learning techniques in important benchmarking problems. This was mainly in the form of improved data representation in supervised learning tasks. Reinforcement learning (RL) is considered the model of choice for problems that involve learning from interaction, where the target is to optimize a long term control strategy or to learn to formulate an optimal policy. Typically these applications involve processing a stream of data coming from different sources, ranging from central massive databases to pervasive smart sensors. RL does not lend itself naturally to deep learning and currently there is no uniformed approach to combine deep learning with reinforcement learning despite good attempts. Examples of important open questions are: How to make the state-action learning process deep? How to make the architecture of an RL system appropriate to deep learning without compromising the interactivity of the system? Etc. Although recently there have been important advances in dealing with these issues, they are still scattered and with no overarching framework that promote then in a well-defined and natural way. This special session will provide a unique platform for researchers from the Deep Learning and Reinforcement Learning communities to share their research experience towards a uniformed Deep Reinforcement Learning (DRL) framework in order to allow this important interdisciplinary branch to take-off on solid grounds. It will focus on the potential benefits of the different approaches to combine RL and DL. The aim is to bring more focus onto the potential of infusing reinforcement learning framework with deep learning capabilities that could allow it to deal more efficiently with current learning applications including but not restricted to online streamed data processing that involves actions.
Topics of interest include, but are not limited to the following:
- Novel DRL algorithms
- Novel DRL Neural architectures
- Novel Reinforcement Learning algorithms with deep representation layer
- Adaptation of existing RL techniques for Deep Learning
- Optimization and convergence proofs for DRL algorithms
- Deeply Hierarchical RL
- DRL architecture and algorithms for Control
- DRL architecture and algorithms for Robotics
- DRL architecture and algorithms for Time Series
- DRL architecture and algorithms for Big Streamed Data Processing
- DRL architecture and algorithms for Governmental Policy Optimization
- Other DRL application
Special Session 2
International Conference Hall I
Bio-Inspired / Energy-Efficient Information Processing:
Theory, Systems, Devices and Applications
Program
Organizers:
Shigeru Nakagawa, IBM Research – Tokyo, Japan
Akira Hirose, The University of Tokyo, Japan
Scope:
Neural networks in the biological brain realize highly flexible and energy efficient information processing based on pattern-processing mechanisms and architectures different from the modern symbol-logic computers. Future computers will employ the principles, structures and devices that integrate these two streams in information processing with a variety of possible hardware. The organizers solicit papers to present the state-of-the-art engineering and technology toward such future energy-efficient computers, especially with bio-inspired features or approaches, for further discussion.
Special Session 3
International Conference Hall III
Whole Brain Architecture:
Toward a Human Like General Purpose Artificial Intelligence
Program
Organizers:
Takashi Omori, Tamagawa University, Japan
Hiroshi Yamakawa, DWANGO Artificial Intelligence Laboratory, Japan
Scope:
The Brain System that realizes high level intelligence is a complex of heterogeneous neural functional elements, not a combination of uniform layered neural network. Those elements must be indispensable functional parts for the human intelligence because an injury of one parts causes somewhat problem for human daily life. Human brain is an existing proof of a possible higher level intelligence beyond a deep learning neural network. Then, it will be a shortest path for building up a human level artificial intelligence to integrate known computational models of brain parts into a computing architecture. So, in this session, the organizers invite papers on a modeling of the brain functional parts, a brain based cognitive architecture and a strategy for integration to present and interact on the state-of-the-art research toward the human like intelligence.
Panes Discussion:
“How can we accelerate study on whole brain architecture?”
Special Session 4
International Conference Hall I
Data-driven approach for extracting latent features from multi-dimensional data
ProgramOrganizers:
Toshiaki Omori, Graduate School of Engineering, Kobe University, Japan
Seiichi Ozawa, Graduate School of Engineering, Kobe University, Japan
Scope:
Due to recent developments in information and measurement technology such as social and sensor networks, the data that we deal with has become very large and high dimensional. For example, sensor networks provide a large amount of multi-dimensional (and multi-modal) data, in which rich information would be potentially latent. Thus, it becomes more important to establish data-driven approach for extracting latent features from such multi-dimensional data. This special session provides a platform for researchers, engineers, scientists and practitioners to present and discuss emerging techniques, principles and applications in extracting latent features from multi-dimensional data by means of data-driven approach. Potential authors/contributors are invited to submit their original and unpublished work in the areas including (but not limited to) the following:
- Bayesian modeling
- Hidden Markov models
- Compressive sensing
- Blind Sensing
- Sparse modeling
- Markov random field models
- Dynamics extraction
- State space modeling
- Dimension reduction
- Visualization
- Clustering
- Human activity classification
- Object tracking
- Action recognition / movie processing
The potential authors/contributors include all researchers who are working in the relevant areas covered by the theme of this Special Session.
Special Session 5
Conference Room III
Topological and Graph based Clustering methods
ProgramOrganizers:
Rushed Kanawati, SPC University Paris 13
Nistor Grozavu, SPC University Paris 13
Scope:
One of the main tasks in the field of high dimensional data analysis and exploration is to compute simplified, usually visual, views of processed data. Clustering and projection are two main methods classically applied to achieve this kind of tasks. Clustering algorithms produce a grouping of data according to a given criterion such that similar data items are grouped together. Projection methods represent data points in a low-dimensional space such that clusters and the metric relationships of the data items are preserved as faithfully as possible. However, in the actual era of big data and connected devices, a lot of datasets are shaped in form of large-scale dynamic attributed graphs. Data is often distributed and is gathered from different heterogeneous sources. New approaches for data clustering and projection are then required. This constitutes the core topic of this special session. We mainly focus on three related issues:
- Topological data clustering approaches.
- Graph clustering methods, including community detection in multi relational (or multiplex) and attributed networks.
- Collaborative and ensemble approaches that allow combining different learning paradigms.
The special session will include a tutorial that covers latest algorithmic advances in these three areas. Submissions are welcomed to all related topics. A non-exhaustive list include:
- Topological clustering methods.
- Consensus clustering.
- Collaborative clustering.
- Diversity analysis.
- Efficient and incremental similarity graph construction.
- Community detection in complex networks.
- Multiplex network analysis and mining.
- Attributed network analysis and mining.
- Applications: recommender systems, data summarization, data visualization.
Technical Tour
The number of applicants has reached the capacity.We plan to conduct a paid technical tour to visit ATR (Advanced Telecommunications Research Institute International) in Kyoto on October 21th, 2016. In ATR, you will visit the following three laboratories:
Development of ATR Exoskeleton Robots: Dr. Jun Morimoto @BRI Experiment Room
We will introduce our newly developed ATR exoskeleton robots. In addition, we will demonstrate how these exoskeleton robots work.
Introduction of Department of Neuroinformatics: Dr. Yukiyasu Kamitani @fMRI Prisma Room
Brain decoding and brain–machine interface
Network BMI system for supporting elderly and handicapped people: Dr. Takayuki Suyama @ BMI House
For supporting elderly and handicapped people to become self-reliant in daily-life environments such as home and clinic, we have developed the network BMI system to control real-world actuators like wheelchairs based on human intention measured by a portable brain measurement system.
After visiting ATR, we will visit Uji city, Byodoin, and the Fushimi Kizakura Memorial Museum. See the following links for details:
Uji city Byodoin KizakuraThe capacity of this technical tour is only 30 and we will accept participants on first-come first served basis. Please register as soon as the registration page is set up. The tour fair is JPY 5,300 (including a lunch box and an admission fee of Byodoin) and should be paid on the ICONIP 2016 registration site.